Our Work
Empowering businesses with data analytics, digital transformation, cybersecurity, and ethical AI solutions.
Claims Analysis
Healthcare Analytics in Claim Analysis focuses on leveraging AI and machine learning techniques to optimize claim processing, enhance reimbursement workflows, and detect anomalies.
Develop AI models to predict claim denials and optimize reimbursement workflows.
Implement anomaly detection algorithms to identify fraudulent claims.
Use NLP to analyze unstructured claim notes and extract actionable insights.
Automate claim categorization using AI-powered classification models.
Build predictive analytics dashboards to monitor claims trends in real time.
Gross Margin Optimization
Gross Margin Optimization utilizes AI and machine learning to forecast financial metrics, streamline cost-reduction strategies, and identify inefficiencies to improve profitability.
Healthcare Analytics
Apply machine learning to forecast revenue and expenses for improved financial planning.
Develop AI-driven simulations to test various cost-reduction strategies.
Use AI to benchmark margins against industry standards and suggest optimization tactics.
Create predictive models to identify high-cost healthcare services and recommend alternatives.
Leverage AI to analyze operational inefficiencies impacting margins.
Social Determinants of Health (SDOH) analytics leverages AI and data-driven approaches to identify, predict, and address health disparities for targeted interventions and improved outcomes
Social Determinants of Health
Use clustering algorithms to segment populations based on SDOH data for tailored interventions.
Build AI models to predict the impact of SDOH factors on patient health outcomes.
Create geospatial analysis tools to map SDOH disparities and prioritize resources.
Implement AI solutions to recommend policy changes based on SDOH trends.
Use predictive analytics to evaluate the effectiveness of community health programs
Homelessness Metrics
Health Program Performance
Homelessness Metrics leverages AI and predictive analytics to identify at-risk individuals, assess housing conditions, and optimize resource allocation for better health outcomes and program effectiveness.
Health Program Performance focuses on using AI and machine learning to monitor key performance indicators, predict outcomes, and optimize program effectiveness through data-driven insights.
Develop AI models to identify high-risk individuals based on homelessness-related data.
Integrate computer vision tools to assess housing conditions for health impact studies.
Create real-time dashboards to monitor homelessness metrics and program effectiveness.
Use predictive models to simulate the impact of housing policies on health outcomes.
Implement AI solutions for optimizing resource allocation for homeless populations.
Build AI-powered dashboards to track KPIs and identify underperforming programs.
Use machine learning to predict the long-term outcomes of health initiatives.
Automate reporting for program performance evaluations with advanced analytics.
Leverage NLP to analyze program feedback and identify areas for improvement.
Develop AI models to simulate program changes and forecast impacts.
KPI Dashboards Development
Leverage AI-driven dashboards and predictive analytics to enhance supply chain performance and proactively identify opportunities for optimization.
o Create AI-driven dashboards with real-time anomaly detection for supply chain metrics.
o Use predictive analytics to forecast supply chain performance trends.
o Build visualization tools that integrate AI insights for proactive decision-making.
o Automate KPI tracking with AI models, reducing manual reporting errors.
o Incorporate AI to suggest optimizations based on KPI patterns and benchmarks.
Supplier Scorecards
Utilize AI and sentiment analysis to evaluate supplier performance, predict risks, and streamline supplier selection for improved procurement outcomes.
Supply Chain & Procurement Analytics
o Implement AI models to assess supplier reliability based on historical performance.
o Use NLP for sentiment analysis on supplier reviews and communications.
o Develop predictive scorecards to forecast supplier performance and risks.
o Automate supplier evaluation processes with AI algorithms for scoring criteria.
o Leverage AI to recommend top-performing suppliers for new projects.
Implement AI models and reinforcement learning to optimize demand forecasting and inventory management for more accurate and efficient supply planning
Demand Planning/Forecasting:
o Build AI models for real-time demand forecasting and inventory optimization.
o Use reinforcement learning to adapt forecasting based on changing market conditions.
o Implement machine learning to predict seasonal demand patterns.
o Automate demand planning with AI, reducing reliance on manual interventions.
o Create visualization tools that incorporate AI forecasts for better planning.
Inventory Management:
Spend Analysis:
Optimize inventory levels and warehouse efficiency using AI-powered tools and predictive analytics to ensure cost-effective stock management.
AI-driven spend analysis and predictive models help identify cost-saving opportunities and enhance budgeting by analyzing spending patterns and contract terms.
o Develop AI tools for real-time stock monitoring and automated restocking alerts.
o Use computer vision to track inventory levels and identify discrepancies.
o Implement machine learning algorithms to optimize warehouse layouts for efficiency.
o Use predictive analytics to forecast inventory needs and reduce excess stock.
o Leverage AI to simulate various inventory strategies for cost reduction
o Use AI to classify and analyze spending patterns for cost-saving opportunities.
o Automate contract analysis with NLP to identify favorable terms and clauses.
o Build AI dashboards to visualize spend categories and suggest optimization areas.
o Use predictive models to forecast spending trends and budget needs.
o Implement machine learning to detect anomalies and flag unnecessary expenses
Employee Engagement
Boost workplace morale and productivity through real-time feedback and AI-driven insights..
Develop AI chatbots to gather real-time employee feedback and insights.
Use sentiment analysis to evaluate feedback trends and recommend initiatives.
Build predictive models to identify disengaged employees and propose interventions.
Automate surveys and analytics to measure and improve employee satisfaction.
Use clustering algorithms to tailor engagement programs to specific employee groups.
Performance Assessment
Empower data-driven growth with AI-enhanced performance evaluations.
HR & People Analytics
Create AI models to analyze performance data and recommend personalized improvement plans.
Automate performance review processes with AI-driven insights.
Use predictive analytics to identify top performers and potential leadership candidates.
Build visualization tools that track performance trends across teams.
Implement AI algorithms to detect patterns in performance issues and suggest solutions.
Drive strategic change with machine learning models that optimize structures and workflows.
Organizational Effectiveness
Use machine learning to simulate changes in organizational structure and their impacts.
Develop predictive models to optimize workflow efficiency and resource allocation.
Automate reporting on organizational KPIs using AI dashboards.
Use clustering to identify inefficiencies in team collaborations and recommend improvements.
Build tools to forecast the effects of new policies on organizational productivity.
Job Satisfaction
Workforce Behavior Analysis
Leverage AI to uncover satisfaction drivers and strengthen employee retention.
Anticipate workforce trends and plan smarter with AI-driven behavioral insights.
Use NLP to analyze employee reviews and uncover key drivers of satisfaction.
Develop AI models to predict turnover risks and recommend retention strategies.
Automate the tracking of satisfaction metrics and suggest actionable improvements.
Build tools to visualize satisfaction trends across departments and job roles.
Use sentiment analysis to evaluate the impact of workplace changes on employee morale.
Use AI to identify trends in absenteeism, productivity, and workforce dynamics.
Build predictive models to forecast workforce needs and optimize hiring plans.
Automate behavior pattern analysis for proactive HR interventions.
Use clustering algorithms to segment employees based on behavioral trends for tailored initiatives.
Implement AI tools to simulate workforce scenarios and plan for future needs.
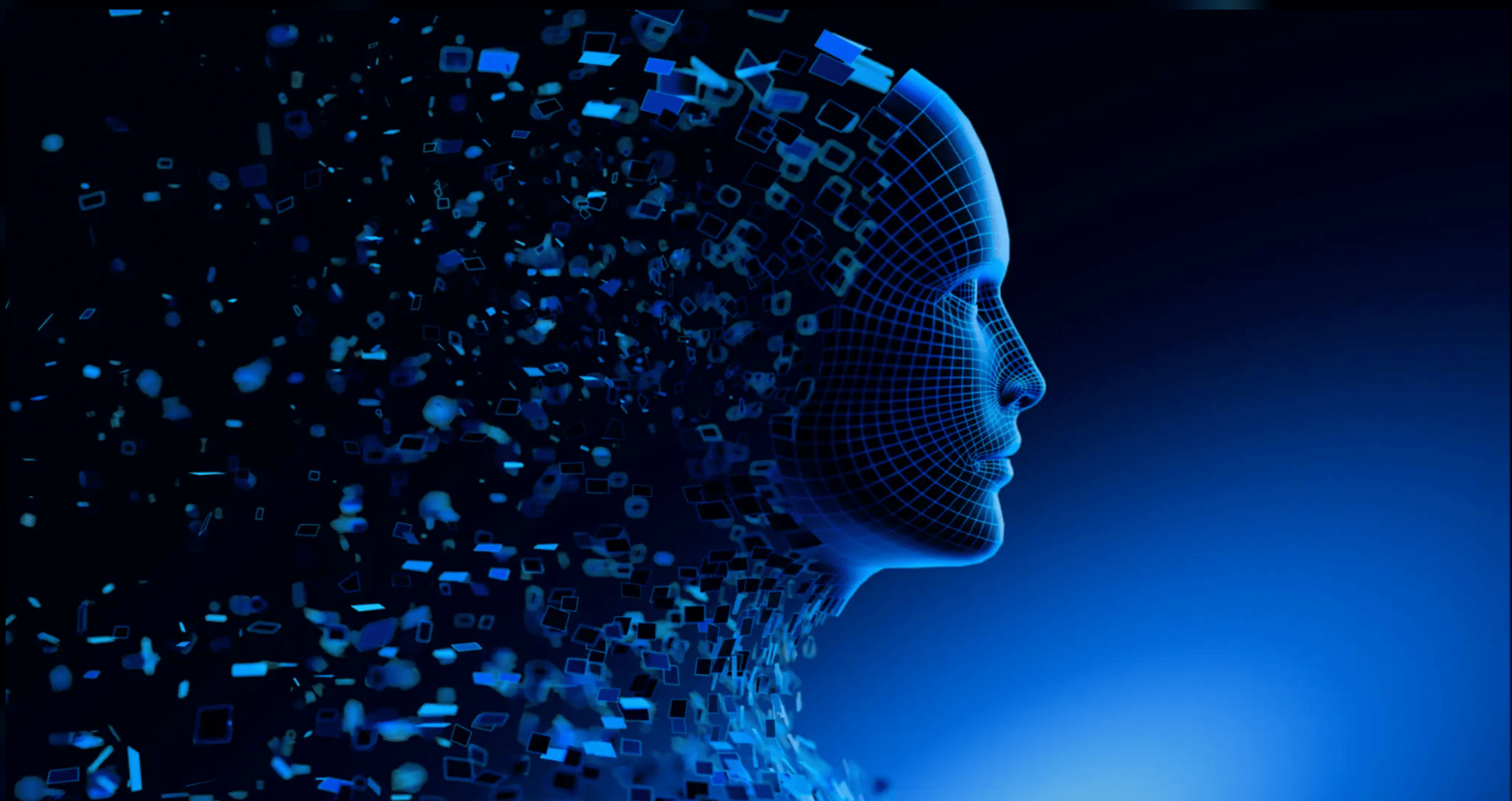
Solutions
Innovative AI solutions for your business needs.
MBM TECH
© 2024. All rights reserved.